How Synthetic Data Can Benefit Your AI-Powered Business in 2024?
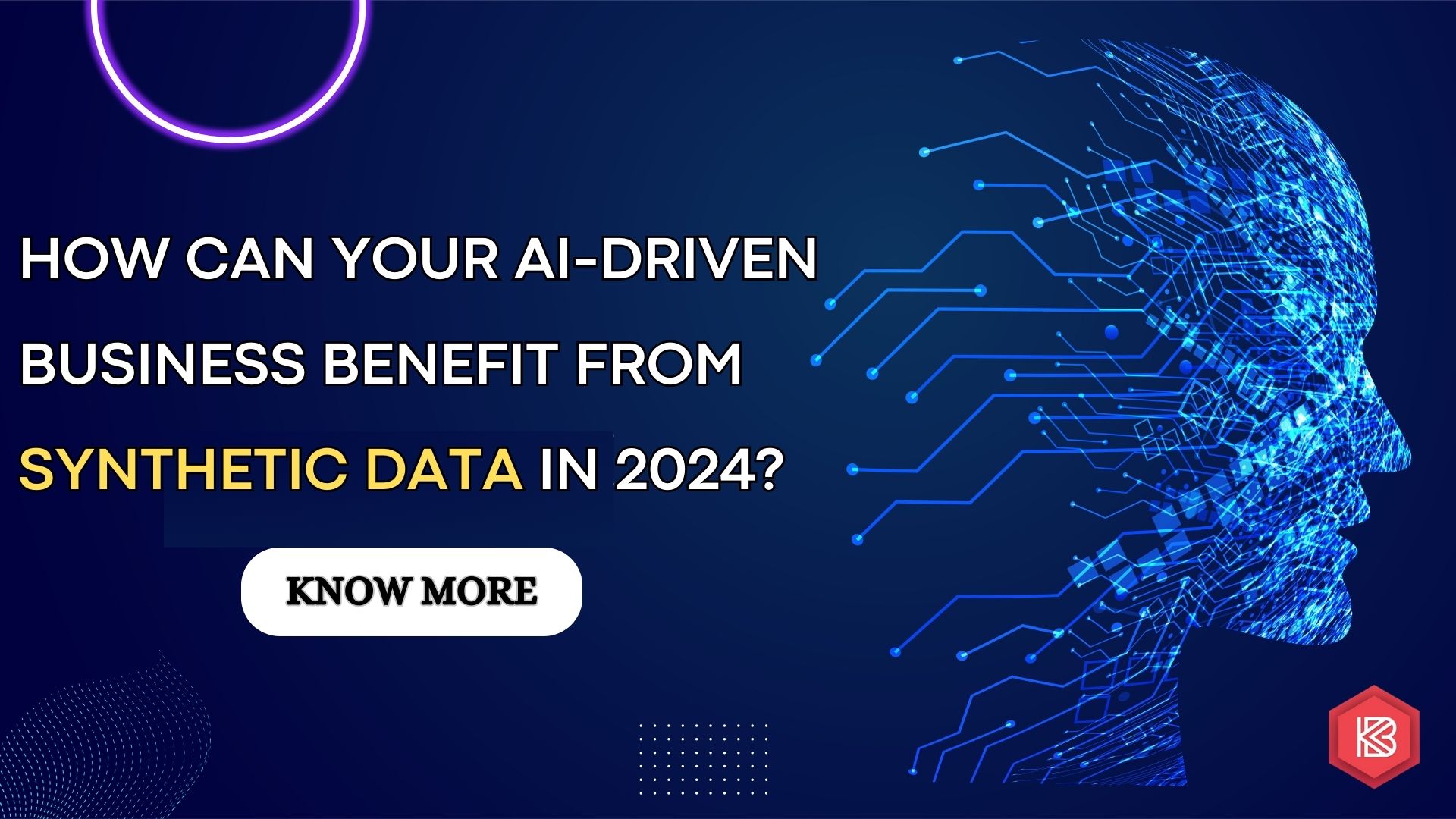
In the swiftly evolving realm of artificial intelligence, a transformative revolution is underway through the utilization of synthetic data. Pioneering companies are now pivotal players, leveraging synthetic data’s potential to redefine the capabilities of machine learning models. This generating synthetic data, artificially generated, serves various purposes such as safeguarding privacy, system testing, and training machine learning algorithms. The efficacy of synthetic data hinges on its quality; hence, its generation is a critical factor. Data that can be reverse-engineered to unveil real information undermines privacy enhancement efforts. Notably, deep learning intersects with synthetic data generation, with algorithms creating synthetic data to enhance other deep learning models.
This guide illuminates the landscape of synthetic data startups, and AI-driven businesses highlighting their avant-garde technologies and innovative methodologies. Witness how these enterprises or AI-driven businesses are reshaping ML models, opening doors to unprecedented possibilities, and driving the AI field toward remarkable advancements.
What is synthetic data actually mean?
Synthetic data, unlike real-world generated information, is artificially crafted through algorithms. Its primary function involves testing operational data sets, validating mathematical models, and training deep learning models. The distinct advantage lies in its ability to alleviate constraints associated with regulated or sensitive data usage. Moreover, it caters to specific requirements unattainable through authentic data sources. Typically utilized in quality assurance and software testing, synthetic datasets offer a tailored approach to data generation, enhancing flexibility and circumventing limitations imposed by sensitive data sources.
What necessitates the use of synthetic data?
Synthetic data proves valuable to businesses for three primary reasons: addressing privacy issues, expediting product testing, and training machine learning algorithms. Stricter data privacy regulations limit how AI-driven businesses handle sensitive information, posing risks of legal repercussions and brand damage upon any inadvertent exposure of personally identifiable customer data.
Consequently, mitigating these privacy concerns ranks as the foremost incentive for companies to embrace synthetic data methods. When dealing with entirely novel products, obtaining relevant data poses challenges, and acquiring human-annotated data incurs significant costs and time. Investing in synthetic data streamlines this process, enabling swift generation and aiding in the development of robust machine-learning models with artificial intelligence.
Beneficial factors of Synthetic Data
Data scientists prioritize accurate patterns, balance, lack of bias, and high quality over the authenticity of data—whether authentic or synthetic. Synthetic data enables enrichment and optimization, providing numerous advantages.
Quality of data – Real-world data, besides being complex and costly to gather, frequently harbors errors, inaccuracies, and biases impacting neural network quality. Synthetic data guarantees superior quality, balance, and diversity. Generated artificially, it adeptly addresses missing values, applies labels, and enhances precision in predictions.
Ability to scale –Training and testing predictive models in machine learning demands vast data quantities, often challenging to acquire at the required scale. Synthetic data serves as a complementary resource, bridging gaps by supplementing real-world data to achieve broader input scales.
User-friendliness – Generating and utilizing synthetic data is typically more straightforward. Gathering real-world data often involves privacy concerns, error filtration, and format conversion. Synthetic data eradicates inaccuracies and duplicates, ensuring uniform formatting and labeling across all data points.
Approaches to Generating Synthetic Data
Generating synthetic data is significantly swifter than manual creation and allows for larger data volumes, ideal for load and performance tests. It’s an essential tool for expediting test cycles and adopting shift-left testing strategies. Yet, distinctions among synthetic data generation methods and technologies affect test data quality, necessitating understanding these differences.
Synthetic data generation systems function based on two fundamentally distinct methods:
A replica of synthetic data emerges by analyzing and profiling production data sources, crafted dynamically to fulfill specific test case needs.
Utilizing deep learning, the initial approach involves modeling and profiling a production database to create a statistically accurate synthetic data replica. This method ensures a secure, private version sans sensitive details, ideal for safe utilization in data analytics and business intelligence scenarios.
Yet, statistically accurate synthetic data isn’t ideal for software testing and quality assurance due to a fundamental drawback: mirroring the limitations of its original database source. Absent patterns or variations in the production data, the synthetic replica will also lack these essential elements.
Employing a synthetic production data replica for testing represents a contemporary shift from traditional Test Data Management (TDM). Unlike conventional TDM systems requiring copying, masking, and refreshing production data, this approach reduces provisioning time. However, missing data variations under TDM diminish overall test data quality and constrain test coverage.
What methods do businesses employ for synthetic data generation?
Businesses opt for various methods like decision trees, deep learning, and iterative proportional fitting to execute data synthesis based on specific requirements and desired data utility. Post-synthesis, evaluating synthetic data involves a utility assessment in two stages. Comparing synthetic data with real data allows businesses to gauge its effectiveness and suitability for the intended purpose of data generation.
Comparisons for broad applications: Assessing parameters like distributions and correlation coefficients between measurements from both datasets.
Assessment of utility considering workload: Assessing output accuracy for a particular use case through analysis conducted on synthetic data.
Selecting the Appropriate AI development company or Synthetic Data Company
Numerous synthetic data creation companies or AI development company and tools exist in the current market. As this partnership is likely a long-term commitment impacting data teams’ software testing and ML model training efficiency, prioritize factors in the following order: Ensuring support for key data generation techniques becomes paramount. Emphasize companies offering these four techniques integrated into their tools during evaluation.
Generative Artificial Intelligence – It learns real-life data distribution to produce synthetic data with a comparable distribution.
Generation based on rules – Creating synthetic datasets using user-defined business rules adds intelligence, maintaining relational integrity by referencing relationships between data elements in the generated data.
Cloning of entities – Gathering data from various source systems within a business entity, like customers, it anonymizes the information to align with privacy regulations. The process involves cloning the entity, and assigning unique identifiers to each clone for distinctiveness.
Masking of data – Replacing sensitive or Personally Identifiable Information (PII) with structurally akin synthetic data is the essence of this process.
Applications of Generative AI Synthetic data
Generative AI Synthetic data serves diverse purposes. High-quality data is essential for machine learning, but real data access might be limited due to privacy issues or insufficient quantity. In such cases, generating synthetic data supplements the model training process. Industries benefit significantly from synthetic data, using it as a complementary resource to enhance machine learning models amidst varying data constraints and privacy concerns.
- Finance and banking sectors
- Healthcare and pharma
- Automotive and manufacturing industries
- Robotics
- Digital marketing and internet advertising
- Intelligence and security companies
The Generative AI Synthetic data’s future
Having explored various synthetic data techniques and benefits, the question arises: Will synthetic data supplant real-world data? Is it the future? Synthetic data exhibits superior scalability and intelligence compared to real data. However, crafting accurate synthetic data demands more effort than AI-driven generation. Achieving precision requires profound AI knowledge and adeptness in managing complex frameworks. Furthermore, the absence of trained models in datasets is essential to avoid skewing, ensuring a closer reflection of reality and addressing existing biases.
Creating a true representation of real-world data necessitates these measures. Synthetic data, designed to emulate reality while mitigating biases, empowers data scientists to accomplish novel feats. Its capacity to enable groundbreaking innovations surpasses what real-world data can offer, indicating a trajectory where synthetic data indeed shapes the future.
Wrapping up
Numerous scenarios arise where synthetic data becomes a remedy for data scarcity or the absence of pertinent information within enterprises. We’ve explored various Generative AI Synthetic data techniques and identified its beneficiaries. Additionally, challenges inherent in working with synthetic data were discussed alongside real-world industry examples where its application is prevalent.
Despite the preference for real data in business decision-making, synthetic data serves as a viable alternative when raw real data becomes unavailable for analysis. Nonetheless, generating synthetic data demands proficient data modeling skills from data scientists. A comprehensive understanding of the actual data and its context is equally vital. This ensures the synthetic data closely mirrors its real counterpart, emphasizing the importance of accuracy and fidelity in the generated data’s representation. To know further details about Generative AI synthetic Data reach out to us.