Unlock Unlimited Potential With Our Advanced Generative AI Services
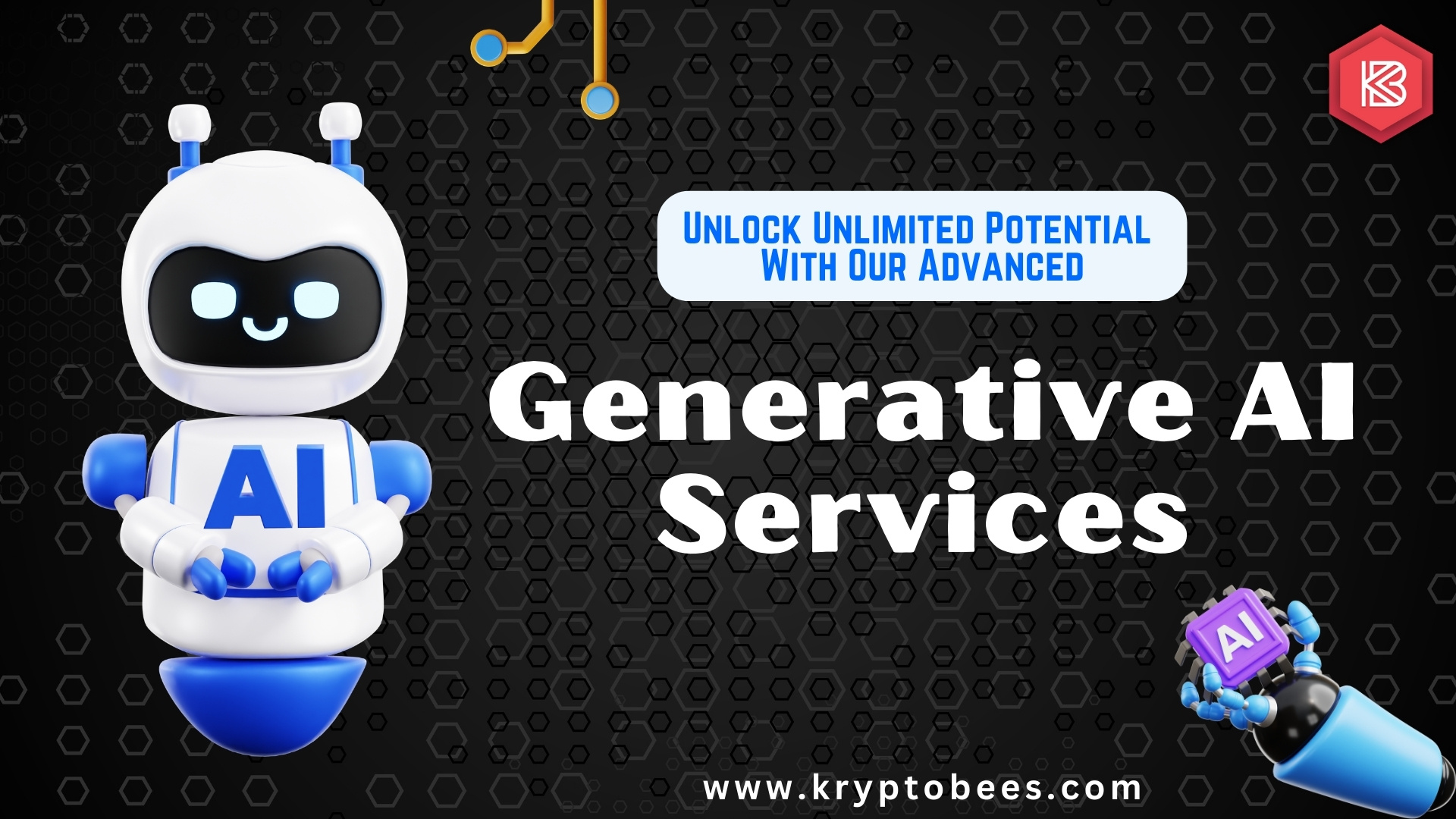
Generative AI, a subset of Artificial Intelligence, has raised the excitement of technologists, researchers as well as the public. As a tool for creation, invention, and human advancement, generative AI has become the major disruptive technology of today that is revolutionizing virtually all spheres of human activity including entertainment, media, business, communication, science, and health facilities. In this blog, we will try to provide detailed information about the AI topic, deepening into the aspects of Generative AI, historical background, and real-world uses, along with the potential benefits recognizable in the context of AI.
Generative AI Meaning?
Generative AI defines algorithms that entail the creation of new work, which may be in writing, images, music, and others. Compared to conventional AI, generative AI entails the creation of new data that resembles the training data that was provided to it and as a result it has prompted new possibilities for creativity and automation.
Generative AI Examples: Generative AI is making an authentic difference in so many areas by making all sorts of new and exciting content. OpenAI has developed GPT-4, A really clever language model that can write text for chatbots including stories and even code. Then there’s DALL-E 2 from OpenAI which can create amazing images based on text descriptions and it is perfect for artists and designers. DeepMind’s AlphaFold is allowing scientists to predict how proteins fold which is extremely crucial for research and medicine. NVIDIA’s GauGAN is shifting simple sketches into realistic images which is awesome for game design and digital art. With Stability AI’s Stable Diffusion, everyone can now create images from text using this open-source tool and making creative AI accessible to all. These examples truly highlight how generative AI is benefiting science, creativity and innovation in so many wonderful ways.
The Evolution Of Generative AI
The Early Foundations Period (1950s-1980s)
The timeline to generative AI started in the middle of the twentieth century with the basic research in Artificial Intelligence. In the 1950s and 1960s, there appeared such theoretical works as the Turing test by Alan Turing. Earlier AI techniques involved rule-based, and a form of symbolic reasoning; this was first illustrated in Joseph Weizenbaum’s ELIZA in 1966, which was a program that mimicked conversation.
Machine Learning existence (1980s to 1990s)
Machine learning appeared later in the 1980s and 1990s in contrast to rule-based learning systems and approaches moving towards learning from data. Neural networks, which copied the structure of the brain, received attention; however, this progress faced many problems because of the limitations of computations and data. During this period back-propagation algorithms were developed which enabled the neural networks to modify the weights and enhance learning.
The Deep Learning Era (2000s – 2010s)
Neural networks began a new wave of acclaim in the 2000s, mainly termed deep learning due to the presence of more layers. The development in computer technology greatly increased the computational capacity and the availability of data which catalyzed the development of AI. An important advancement in AI was GANs took place in the 2010s.
Transformer Models and NLP (2017 – 2020)
The cross-attention or self-attention mechanism was founded in 2017 by introducing the transformer models for NLP. The transformers with their attention mechanisms provided better ways to manage dependencies in the data, which significantly boosted the generative AI in language capabilities.
Recent Development (2021- Till Now)
Generative AI has been thus becoming more and more sophisticated in recent years due to continuous academic work and industrial adaptations. In addition to that, the newly introduced techniques, including reinforcement learning, transfer learning, and unsupervised learning have been also incorporated with the generative model which can be regarded as significant improvements to the current generative models and show more significant potential for future improvements.
Key Aspects Of Generative AI
Neural Networks and Deep Learning: Generative AI basis can be traced to neural networks which have undergone development from as early as the 1940s. Deep learning, a subcategory of machine learning, uses neural networks with multiple layers for learning increasingly complex representations of the data.
Generative Adversarial Networks (GANs): GANs were proposed by Ian Goodfellow in 2014 and consist of two neural networks: a generator and a discriminator that work in pairs. The generator generates more data and the discriminator checks the validity of the data produced by the generator. This adversarial process implies the generation of highly realistic data.
Variational Autoencoders (VAEs): VAEs, which are also another powerful generative model, were also proposed at roughly the same time as GANs. They map their input data into the latent, usually less-dimensional space, then map it back to the output space to produce data samples similar to the input.
Transformer Models: The transformer-based models dramatically shifted the direction of the natural language processing. It said these models could produce logical and contextually accurate prose, including for communication devices, auto-writing, and language interpreting.
Benefits Of Generative AI
Generative AI offers several valuable benefits:
- Automated Content Production: It helps businesses to create high-quality content in bulk thus making it efficient, cheap, fast, and convenient.
- Improved Customer Experience: Recommendations and product suggestions are built individually by generative models, increasing the customers’ experience and their interaction level.
- Cost and Time Efficiency: Businesses can automatically generate content, save a great deal of cash on labor, and shorten product development cycles.
- Task Automation: Generative AI is to reduce repetitive and manual work in the HR department across core working areas such as data entry, image, video editing, and report generation, among others, by automating such processes free of charge.
- Data Analysis: Identifying patterns from big volumes of data, will enable the firms to know how their customers behave, potential markets, and opportunities within the market, and hence proper strategies and decisions will be enhanced.
- Personalization: Generative AI returns good value at scale as it scientifically creates customized recommendations, and products to improve sales.
- Customization: This enables the creation of customized products or services where the business entity is in a position to offer specific customers what they want.
Top 5 Growing Generative AI Sectors
- Finance: Generative AI is applied by financial institutions in decision-making for efficiency in financial forecasting and to manage risks. Analyzing historical information and other factors, AI models can provide valuable forecasts to optimize the financial planning of businesses. In the same way, generative AI also uses algorithms to detect fraudulent transactions to curb the scramble. It forecasts market conditions which help the banks in intervention strategies. Moreover, generative AI predicts market opportunities and thus can help enterprises with investments and risks.
- Supply Chain Optimization: Dynamic generative AI enhances the ability to forecast future demand for products, thus improving operations in the supply chain. This is well managed by generative AI since it predicts customer demand, recognizes disturbances en route, and even the best route. This translates to cost reduction and generally improved operational efficiency among the firms.
- Customer Support: One more way that generative AI can be really helpful is by improving customers’ experience due to a personal approach. Customers’ data and behavior in their interactions produce an AI model that makes recommendations, products, and real-time responses. This enhances the image of organizations and improves the total quality of customers.
- Healthcare: Generative AI models diagnose and interpret medical imagery and descriptions, patients’ electronic health records, and clinical study information to assist healthcare workers in reaching better diagnostic conclusions as well as devise more effective treatment plans. Society enables the usage of generative AI to come up with drug candidates for Clinical trials through simulations. It also allows the researchers to predict the changes in the gene expression, thus, fostering the process of gene therapy. Furthermore, it enhances medical image analysis to increase the correct likely diagnosis of diseases or conditions.
- Gaming: Gaming helps create new levels, characters, or any other element that may be required to be added to the video games. They assist in creating the design elements, which could be avatars or any other graphic content. Generative models can also be used in making educational simulations such as the flight simulator that many flying institutions use.
The Future Of Generative AI
- Advancements in AI Research: It is possible in the future with advancing studies in AI alongside machine learning to develop new methods that improve generative AI. It is safe to expect increases in quality and variety, as well as gains in efficiency of any generated content. More research works and organizations are paying attention to eliminating biases, enhancing model interpretation, and making generative AI technologies more ethical.
- Integration with Other Technologies: Generative AI will be incorporated with other trends progressively including AR, VR, and IoT. Such integration will lead to the manufacturing and deployment of highly engaging experiences and new solutions in many fields.
- Education and Workforce Transformation: Generative AI will change the nature of work and generate new jobs as well as alter the nature of many existing jobs. Education systems will require certain changes to address these issues and integrate the methods of AI literacy education and training to prepare the population for the future demand of the job market. Generative AI is also growing into related areas such as music generation, drug design, and pharma–individualized medicine. There are future works underway on multimodal models that can work and generate multiple kinds of input and data at the same time and produce better and more synchronized AI systems.
Conclusion
Generative AI is a great advancement in artificial intelligence that provides the advantage of new and creative thinking. It is used in such diverse fields as art, entertainment, health care, and business. Selecting Kryptobees as the AI Development Company to develop your AI models indicates you are going with the best, and most reliable Company around. Our experts create highly customized artificial intelligence solutions adjusted to your company’s specifics. Machine learning, natural language processing, computer vision, predictive analytics and every service associated with AI are completely inclusive within us. As the leading AI Development Company, we offer crisp communication both internally and externally, and a client-centered orientation to ensure that your vision is implemented accurately and promptly. The portfolio of successful projects shows that our company can help various industries achieve substantial change. Select Kryptobees and achieve the advanced capabilities of artificial intelligence for your business. There are great expectations for generative AI in the future, it is expected that AI systems will be able to create a better world and a better tomorrow.